6 min read
An Introduction to DuckDB
This is a summary of a book chapter from DuckDB in Action, published by Manning. Download the complete book for free to read the complete chapter.
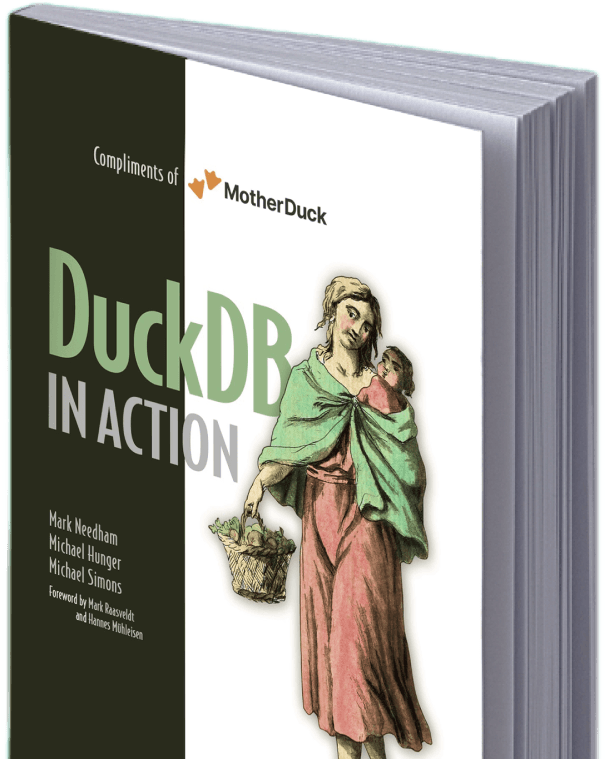
1.1 What is DuckDB?
DuckDB is a modern, embedded analytics database designed for efficient processing and querying of gigabytes of data from various sources. Unlike traditional client-server databases, DuckDB operates within the same process as your application or notebook, eliminating network overhead and simplifying deployment. Developed by database researchers at Centrum Wiskunde & Informatica (CWI), DuckDB boasts a robust ecosystem supported by DuckDB Labs, the non-profit DuckDB Foundation, and a thriving community on platforms like Discord and GitHub. Its ability to handle diverse data formats such as CSV, JSON, Parquet, and Apache Arrow, coupled with its integration with databases like MySQL, SQLite, and Postgres, makes it a versatile tool for data professionals.
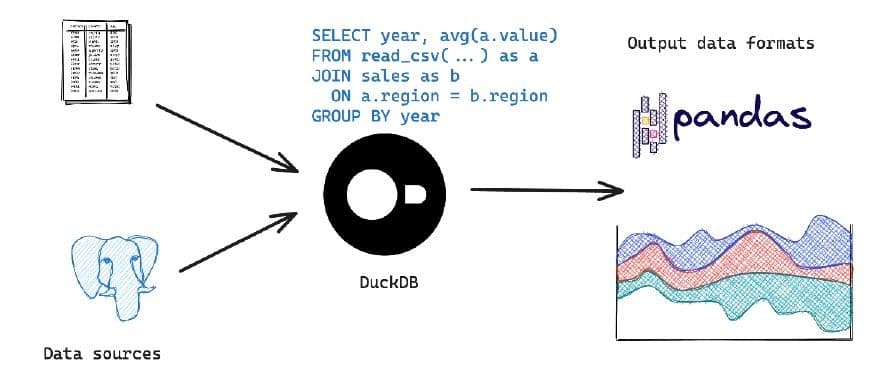
1.2 Why should you care about DuckDB?
DuckDB simplifies data analytics by providing a fast and efficient way to process substantial datasets locally without the complexities of distributed computing frameworks or cloud-based data warehouses. This translates to faster analysis, reduced costs, and streamlined workflows. Unlike traditional data manipulation libraries, DuckDB's SQL-based approach and columnar data processing engine enable it to handle larger data volumes with superior performance. Moreover, DuckDB's SQL dialect extends the ANSI standard with features like simplified SELECT statements, PIVOT and UNPIVOT operations, and support for complex nested data through the STRUCT data type, enhancing its analytical capabilities.
1.3 When should you use DuckDB?
DuckDB is suitable for analytical tasks involving structured data up to a few hundred gigabytes, where SQL-based analysis and transformation are required. Its ability to handle wide tables with numerous columns and large tables with extensive rows makes it ideal for diverse analytical workloads. DuckDB excels in scenarios involving analysis of log files, personal data analysis on edge devices, and pre-processing data for machine learning, especially when data privacy is paramount. Its efficient integration with Python and R data science environments, like pandas and Polars DataFrames, further extends its utility for data scientists and analysts.
1.4 When should you not use DuckDB?
DuckDB is not designed for applications requiring concurrent write access or transactional integrity due to its focus on analytical workloads. While it supports spilling data to disk for processing beyond available memory, it's optimized for datasets fitting primarily in RAM, making it unsuitable for terabyte-scale data processing. Similarly, DuckDB is not a streaming database and lacks built-in support for continuous data ingestion or real-time analytics on constantly updating data streams. In enterprise environments with complex data ecosystems and massive data volumes, specialized tools might be more appropriate.
1.5 Use cases
DuckDB's versatility shines in numerous applications. Its embeddable nature makes it ideal for integration into cloud, mobile, desktop, and command-line applications, enabling efficient analytics directly where data resides. This proves particularly valuable for privacy-sensitive data analysis on personal devices, reducing reliance on data transfer and cloud processing. In data science, DuckDB streamlines data preparation, exploration, and feature engineering, offering a performance boost over traditional DataFrame libraries. Its ability to process data locally and efficiently positions it as a cost-effective alternative to cloud-based analytics services for tasks like log file analysis.
1.6 Where does DuckDB fit in?
DuckDB serves as a powerful engine for analyzing and transforming data residing in various formats like CSV, Parquet, JSON, or databases such as PostgreSQL and SQLite. It can be used transiently for data manipulation or to create persistent tables for analytical queries. DuckDB's ability to handle nested data, perform data cleaning, and reshape data structures makes it a valuable asset in data pipelines. Its efficient querying capabilities, combined with data visualization tools, enable users to gain insights from data and communicate findings effectively.
1.7 Steps of the data processing flow
DuckDB's data processing flow involves a series of steps: loading data from various sources and formats, understanding the data structure, developing SQL queries for analysis, and utilizing the results. Its support for diverse data formats, including CSV, JSON, Parquet, and Apache Arrow, simplifies data ingestion. DuckDB's ability to infer schema information and handle nested data structures streamlines data preparation. Its SQL dialect, along with features like common table expressions and window functions, facilitates complex data analysis. Finally, results can be exported to various formats, visualized, or used in downstream applications.
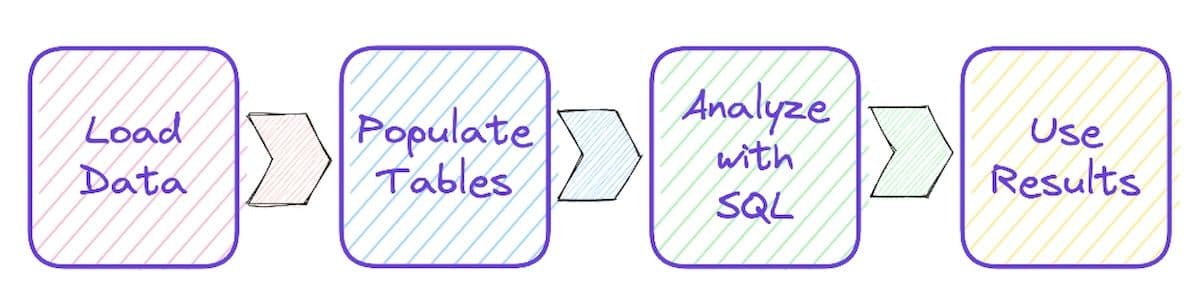
1.7.1 Data formats and sources
DuckDB's strength lies in its ability to handle a multitude of data formats and sources with minimal configuration. It seamlessly integrates with common data formats like CSV, JSON, and Parquet, enabling efficient loading and processing. DuckDB's support for Apache Arrow, a columnar in-memory data format, further enhances performance by minimizing data serialization and deserialization overhead. Additionally, it can directly query data from cloud storage services like AWS S3 and Google Cloud Storage, reducing data transfer costs and simplifying data pipelines.
1.7.2 Data structures
DuckDB supports a rich set of data structures, extending beyond traditional data types like integers, floats, and strings. Its support for lists, maps, structs, and enums enables efficient handling of complex data. Lists and arrays allow storing multiple values of the same data type, while maps provide a key-value storage mechanism. Structs enable defining custom data types with named fields, enhancing data organization and readability. Enums allow representing a fixed set of values, improving data validation and code clarity.
1.7.3 Developing the SQL
DuckDB employs a familiar SQL dialect, making it accessible to users with SQL experience. Starting with basic queries to understand the data's shape and distribution, users can progressively build more complex analytical queries. DuckDB's support for common table expressions (CTEs) improves query readability and modularity. Its rich set of aggregate functions, including statistical functions and window functions, enables sophisticated data analysis. The ability to visualize query results directly within DuckDB using bar charts enhances data exploration and understanding.
1.7.4 Use or process the results
Once data is processed and analyzed using DuckDB, the results can be utilized in various ways. DuckDB allows exporting query results to different formats, including CSV, JSON, and Parquet, facilitating data sharing and integration with other tools. Results can also be persisted in DuckDB tables for further analysis or used to create materialized views for efficient querying of pre-computed data. DuckDB's integration with Python and R enables seamless visualization of results using libraries like matplotlib, ggplot2, and d3.js, enhancing data storytelling and communication.
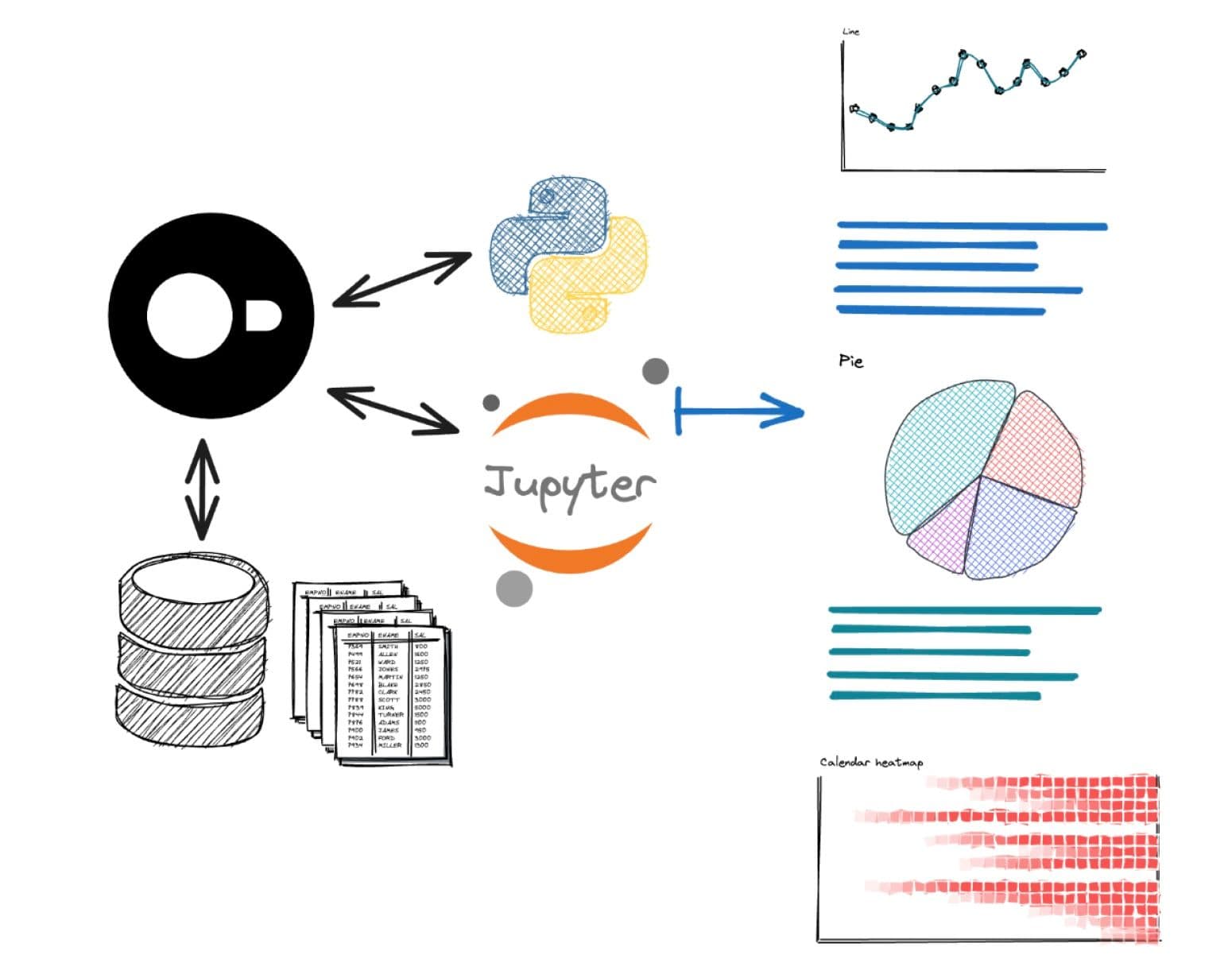
1.8 Summary
DuckDB emerges as a powerful in-memory analytical database, excelling in processing and querying gigabytes of data efficiently. Its support for an extended SQL dialect, diverse data formats, and seamless integration with popular programming languages makes it a versatile tool for data professionals. DuckDB's ability to handle complex data structures, perform efficient aggregations, and visualize results directly enhances its analytical capabilities. Its ease of use, performance, and versatility position it as a valuable asset for various data-intensive tasks, from ad-hoc analysis to building data pipelines.
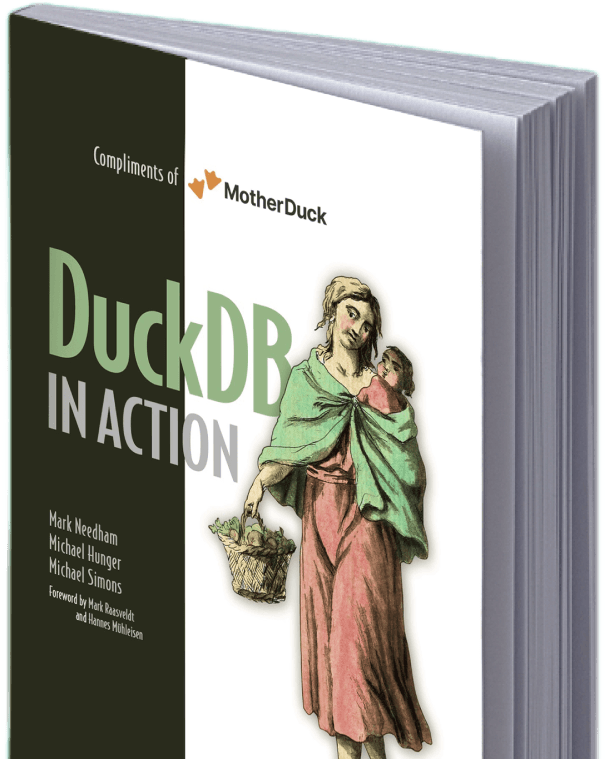