Small Data is bigger (and hotter đ„) than ever
2024/10/19 - 12 min read
BYIn late September, we held the first Small Data SF with our friends at Turso and Ollama, a two-day, in-person event featuring hands-on workshops and technical talks and sessions.
With more than 250 attendees and a packed agenda, we gathered in San Francisco to learn how to take a smaller, more pragmatic approach to simplifying our work. We mingled, shared ideas, started conversations with our awesome community, and listened to over 20 speakers with novel outlooks on this topic.
Letâs take a moment to recap what we learned.
But first, here are a few stats about the event itself:
- 14 keynote and technical sessions
- 1 practitioner panel of data and AI leaders with in-the-trenches experience
- 7 hands-on, instructor-led workshops
- 80+ net promoter score (NPS), which likely means weâll be doing this again đ
Emerging Trends in Small Data
âI think Small Data is a very important trendâŠmaybe the most important trend right now.â â George Fraser, Fivetran Founder and CEO
Small Data is mighty, and it isnât just about the Small Data Manifesto.
Our top learnings and insights from Small Data SF 2024 focus on several key themes -
- Real Data Volumes Arenât as Big as we Thought
- Agency Matters: The Future is Flexible and Multi-Engine
- The True Cost of Big Data: Time, Money, and Complexity
- Local-First, Cloud-Second Architectures
- The Power of Smart AI and Local Models
- 'Hot Dataâ Rising: A Return to Joyful Data Workflows
The Case for Real Data
âHow big are your actual queries? The fact that you've got a Petabyte of logs sitting on disk doesn't matter if all you're looking at is the last seven days.â - Jordan Tigani
Thanks to the separation of storage and compute, working datasets tend to be much smaller than overall data volumes, and tools like DuckDB have been pivotal in driving the shift in focus toward processing not-so-big data volumes efficiently.
While MotherDuck founder and CEO Jordan Tigani highlighted how businesses often deal with datasets that donât require the complexity and cost overhead of big data systems to deliver business insights, others, like Benn Stancil, urged the audience to innovate and build better solutions to help users interpret and derive meaning out of smaller datasets.
Lindsay Murphy, Head of Data at Hiive, took yet another approach to the topic of real data and implored the audience to think inside the box and use constraints to drive innovation and prioritization over the endless pursuit of more data, dashboards, and trashboards for the sake of it.
Finally, a broader theme from the talks centered on our actual data workflows and use cases. To underscore the importance of data ingestion, which modern benchmarks fail to capture effectively, Fivetran CEO George Fraser shared that about 30% of most analytics workloads can be attributed to data ingest.
Agency Matters: The Future is Flexible and Multi-Engine
â...I do believe that the future will be a multi-engine data stack where we will choose different tools and how to execute based on the scale of the data, but hopefully, our APIs and workflows will become more and more common so that we can work locally and deploy anywhere.â - Wes McKinney
With the rise of multi-engine architectures enabled by the emergence of the data lakehouse architecture, flexibility is being taken to new heights without sacrificing costs or efficiency. Speakers including Wes McKinney, Posit PBC Principal Architect and Co-founder of Apache Arrow and Pandas, retraced the history of modern hardware and data warehousing that has given way to the emergence of the Small Data ethos. In the 2010s, we collectively realized a need for interoperable table and columnar data formats that can be used portably across different programming languages and processing engines.
DuckDB Labsâ Richard Wesley also highlighted the provenance of computing that led to the creation of DuckDB by recounting his own journey in software and computing. He emphasized the ability of great software to integrate and talk to other tools and systems with connectors and data transformation. As the glue that ties together this emerging ecosystem, DuckDB has notably helped make way for new tools and ways of working.
âEverything's much more pluggable than it used to be. You used to have to pick a tool, and that was the tool you usedâŠso if you had a problem that was untenable with cheaper tools or whatever, then that was the tool you ended up using for everything because you were locked into your overall stackâŠNow, we [have] the option to compose our approaches to different problems.â - James Winegar, CorrDyn CEO
Big Data is Costly and Complex
âWe were promised these previously unimagined insightsâŠand instead we got these directional vibes, where you look at the chart, and you're like, itâs âup-ish,â I don't know.â - Benn Stancil
The 'cloud tax' and inflated processing costs in incumbent platforms underscore the inefficiencies of big data infrastructure that have sparked a shift toward more cost-efficient solutions.
Several speakers, including Benn Stancil and Turso Co-founder and CEO Glauber Costa, discussed how big data systems are often overengineered to meet the needs of most businesses, who are looking for insights and support with interpreting their normal-sized data.
In a world where single nodes and scaling out are becoming a more standard architectural pattern, Glauberâs proposal to make per-user tenancy a more widespread model is highly appealing thanks to its flexibility and simplicity. By giving each user their own database, developers wonât have to worry about things like role-level security because the database becomes their access boundary and eliminates the need for caching.
Gaurav Saxena, Principal Engineer at Amazon Redshift and author of 'Why TPC is Not Enough', shed some light on the issue of overengineered systems by discussing the inadequacies of TPC benchmarks in providing effective database evaluations and recommendations for customers based on their real needs. His analysis of of the Redset dataset from Amazon Redshift customers provides insights into query patterns and workload distributions that TPC benchmarks fail to capture. Because databases face a long tail of complex, resource-intensive queries, it is important for them to manage short, repetitive, bursty queries and continuous data ingestion and transformation.
From an end user standpoint, discussions of scalable, interactive data visualizations by University of Washington PhD student Junran Yang also highlighted the need for better ways to interact with data. Both academia and industry are focusing on simplifying data exploration to make insights more accessible and actionable for users. Scalability and interactivity that match user expectations are key to creating practical visualization solutions that use emerging technologies to simplify the complexities of Big Data.
Together, these talks point to a future where simplicity, cost-efficiency, and flexibility dominate the data landscape, with tools and systems tailored to specific needs without sacrificing performance.
Local-First, Cloud-Second Architectures
âIf you have an application built in this local first way, you can run it without the cloud. You can run it offline for a while and then sync later. Even if the cloud goes away or the company goes out of business, as long as you still have the application and your data, you can keep it running. - SĂžren Brammer Schmidt, Prisma Founder and CEO
Our technological evolution in recent years has focused on modular, scalable systems that can adapt to changing demands. Systems that allow for local development with remote deployment offer better cost controls and performance. The re-emergence of single-node systems and the adaptability of platforms like DuckDB further emphasize and demonstrate this growing trend.
SĂžren Brammer Schmidtâs discussion on local-first architecture and its potential to revolutionize software development mirrors the broader move towards decentralization and moving the database to the client, close to end users. This trend aligns with a wider theme from other talks around smaller, more efficient data systems that reduce the reliance on cloud infrastructure.
Chris Laffra picked up a different angle on this topic and introduced the audience to his new project, PySheets, a local-first open-source project that embeds Excel in Python to reimagine data exploration through graph dependency visualization within spreadsheets while running in the web browser. Inspired by the belief that conventional tools like Jupyter Notebooks and Python in Excel are limiting, PySheets enables intuitive, offline data manipulation without reliance on cloud services.
Smart AI and Local Models
âThese small models only have maybe 0.5 to 70 billion parameters. They are only a few gigabytes in size, which means they definitely fit on your laptop - heck, they even fit on a phone, and they run on ordinary hardware, so you don't need these really expensive, hard-to-buy clusters of GPUs all wired up in a special way to run them. You can actually run them right here on your existing computer.â - Jeff Morgan, Ollama Founder
Itâs no secret that AI and machine learning are significantly reshaping content creation, data analysis, and user engagement. Jeff Morgan, a founder of the open-source project Ollama, highlighted its power by demonstrating its ability to run LLMs and Small Language Models locally on consumer-grade laptops. He emphasized the capabilities of faster and more versatile small AI models due to their reduced parameter size and suitability for local operation without network dependency. While small models are not suitable for every task, they provide a unique complement to larger, cloud-based models and offer better performance and flexibility for tailored use cases.
Later in the day, Buzzfeed Head of Data Science, AI, and Analytics Gilad Lotan showcased how LLMs and AI tools have been integrated into their generative content systems to enable them to create a participatory style of commenting on newsworthy stories, while Langchain GTM Lead Julia Schottenstein discussed how Langchainâs langraph framework can balance flexibility with reliability to turn traditional directed acyclic graphs (DAGs) into directed cyclic graphs, or agent-based systems where LLMs dynamically control application workflows to allow for a more flexible and iterative workflow.
Inspired by all the excitement around small AI and local models, we recently decided to jump into the fray here at MotherDuck by embedding a large language model inside SQL.
Hot Data Rising: The Simple Joys of Small Data
âWhen I think about Small Data, it's that layer of data you're actually using and working with. It equates to hot data: the data thatâs driving business value and decision-making, not whatâs sitting in storage.â - Celina Wong, Data Culture CEO
The key driver of cost and performance efficiency in Big Data systems with separated storage and compute is the size of the hot data. More data doesnât mean better results, and we closed Small Data SF with a spirited panel discussion on data minimalism moderated by Ravit Jain to highlight what it takes to deliver real business value for the 99% of organizations that donât have Big Data.
Even in a Small Data environment, organizations still have considerable stakeholder demands for insights and data-driven decision-making. Josh Wills highlighted that unlike the era of Big Data, Small Data is focused on the power and importance of individual machines. Now that laptops are powerful, workloads and use cases that once defaulted to the cloud can be executed locally, in full or in part, on a single machine.
âWe care about individual machines, we are excited about the potential, and we are writing software to optimize the potential of a single machine. We're not just focused on lots and lots of dumb individual machines anymore.â - Josh Wills, Technical Staff at DatologyAI
Jake Thomas, Data Foundations Manager at Okta, also touched on the need to optimize for cost efficiency while avoiding the lure of over-engineering or over-provisioning your infrastructure as a defensive strategy against edge-case scenarios that may never come to pass. For 80-90% of everyday insights and analytics use cases, we only work with hot data, the thin slice of data containing the value you need to make business decisions.
Shouldnât we return to making our data work for us? What happened to making data workflows simple, scalable, and fun? Or, in the words of Marie Kondo: If it doesnât spark joy, do you need it?
Small data and AI is more valuable than you think.
Celebrating the Small Data Community
The most exciting part of Small Data SF wasnât just the talks: It was the group of people who came together to build this movement. On site, I quickly lost track of the number of people who flagged me down to ask, âHow did you get such good attendees? When is the next one? How do I get involved?â
Frankly, I canât take credit for this. You all decided to show up and bring this event to life by making it yours. And if you didnât make it this time, I hope it has piqued your curiosity and sparked something in you to find out more so you can think small, develop locally, and ship joyfully! We see you, and weâre hard at work thinking about opportunities to get more people involved.
See you in 2025?
Weâre hard at work putting the finishing touches on recordings of the talks, and weâre scheming up more plans to release these and share them online and potentially in some major cities near you. Stay tuned.
Something small is happening, and it has only just begun. The overwhelming feedback we have received points to one key theme: The people want more opportunities to come together around Small Data!
Thank you to our attendees, speakers, sponsors, and co-organizers who joined us from around the world and to our extended event production team, vendors, and the MotherDuck team for being on the ground to engage with this small but mighty community. We could not have done this without you, and we look forward to seeing you at upcoming events.
Small Data SF would not have been possible without our friends at Turso and Ollama and our generous sponsors: Cloudflare, dltHub, Evidence, Omni, Outerbase, Posit, Tigris Data, and Essence. Thank you for your support in bringing the very first Small Data SF to life!
CONTENT
- Emerging Trends in Small Data
- The Case for Real Data
- Agency Matters: The Future is Flexible and Multi-Engine
- Big Data is Costly and Complex
- Local-First, Cloud-Second Architectures
- Smart AI and Local Models
- Hot Data Rising: The Simple Joys of Small Data
Start using MotherDuck now!
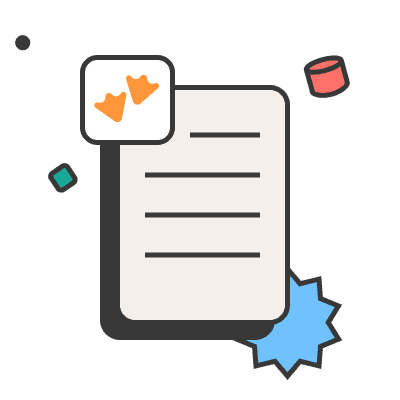